To view in your browser and/or download the article, click on the PDF below.
This explainer article defines linking measures and describes:
- Why linking measures are useful and even necessary in explaining research to lay audiences
- How to distinguish between linking and non-linking measures
- Methods for depicting outcomes that enhance social comprehension
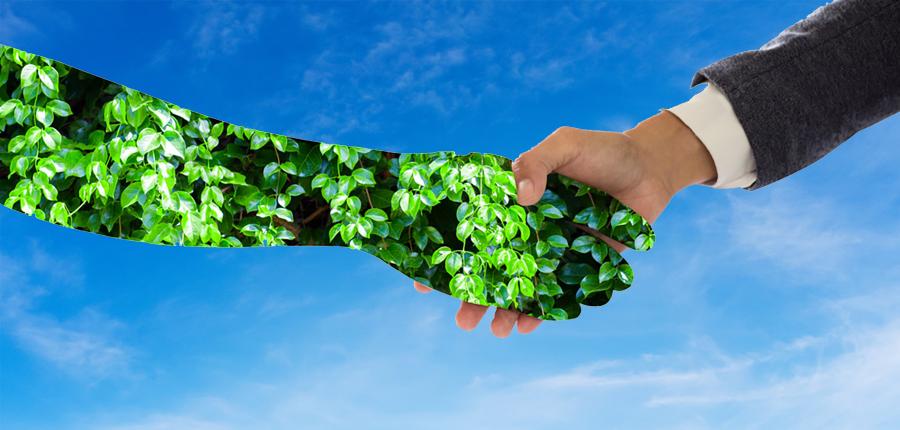
What Is a Linking Measure?
Socio-environmental analysis requires ecological and social researchers to integrate or link their models and data. Certain measures, outcomes, and indicators facilitate this integration—what we refer to here as “linking measures.” Linking measures are important boundary objects.1 In a nutshell, they are biophysical measures that facilitate social evaluation and are meaningful and easily interpretable outside the natural science research community.2 More technically, they can be thought of as natural science outcome measures (e.g., dependent variables) that are useful input measures (e.g., independent variables) for social science research.
What Makes a Biophysical Measure “Useful for Social Science Research”?
The basic test is: can a lay person understand the measure and its importance to their or society’s well-being? In S-E research, social science is largely about why nature matters to people, what people care most about, and how nature can be managed and protected to align with social values. When lay audiences (and social scientists) don’t understand why or how a biophysical outcome matters to people, a big barrier is erected between the natural and social elements of the research.3
For example, if an ecological study reports a change in riverine nitrogen levels, that has inherent importance and meaning to the natural science community. But it may have little or no meaning to social audiences and researchers. Instead, the goal would be to translate nitrogen levels into outcome measures reflecting fish abundance, or water clarity and odor. These kinds of outcomes not only explain why N is important, but also facilitate social analysis about how much it matters and what people would like to do about it.
Hints to help distinguish between linking and non-linking measures: |
---|
|
We encourage S-E researchers to think about linking measures as useful (or even necessary) boundary objects for integrated natural and social science research. Identification of linking measures can also stimulate its own research questions. For example, if quantifiable linking measures seem unavailable, that suggests a need for studies that quantitatively relate existing measures to new kinds of biophysical outcomes, e.g., oyster catch, seagrass cover, etc.
Which Types of Biophysical Measures Are Easily Translatable as Linking Measures and Are There methods for finding them?
Effective linking measures are often biophysical measures, such as natural resource quantities, qualities, features, and conditions. Some are easier to measure than others; e.g., location-specific species abundance predictions are more difficult to measure than a species’ presence vs. absence. Over the last decade, ecologists and social scientists have collaborated on lists and taxonomies of linking measures and ways to identify measures most useful to S-E analysis.4, 5 It is also important to note that there is not a single, short list of linking measures. Rather, linking measures reflect the myriad ways in which people interact with and value nature.
One particularly useful method is to depict an ecological system as a system of production, with a network of linked biophysical drivers, stressors, and outcomes.6 Researchers can use system diagrams to identify outcomes that make good “social comprehension boundary objects.” In general, it is useful to think causally, by taking a familiar measure and asking: is this measure an input to, or driver of, other biophysical features that would seem tangibly important to someone like my next-door neighbor? If your focus is a benthic macroinvertebrate biomarker (not a good linking measure), for example, relate it to a swimmer’s risk of waterborne illness or chance of trout being present.
a more understandable measure – “fishing quality”).
Credit: The Ecological Society of America
Examples of Linking Measure Research Questions
- Would the meaning and importance of your research’s biophysical outcomes be clear to lay audiences (and your social science research partners)?
- Is there existing biophysical research that would allow you to connect your outcome measure to other biophysical outcomes measures that are more socially meaningful?
- Is brand new research needed to quantify such connections?
Key Takeaways
- Linking measures are key boundary objects in S-E research that facilitate the integration of natural and social science research components.
- Biophysical outcome measures that involve technical or scientific jargon do not make good linking measures.
- Linking measures tend to be biophysical outcomes that people can see, smell, hear, taste, or touch.
- There is not a single, short set of linking measures appropriate to all S-E research, though examples from the literature may provide useful examples.
1Boundary objects can be described as concepts, images, or other items that facilitate communication across and between different groups.
2James Boyd, Paul Ringold, Alan Krupnick, Robert Johnston, Matt Weber, and Kim Hall. “Ecosystem Services Indicators: Improving the Linkage Between Biophysical and Economic Analyses,” International Review of Environmental and Resource Economics Vol. 8, No. 3–4 (2016): 359-443.
3Schiller, A., C. Hunsaker, M. A. Kane, A. K. Wolfe, V. H. Dale, G. W. Suter II, C. S. Russell, G. Pion, M. H. Jensen, and V. C. Konar. “Communicating Ecological Indicators to Decision Makers and the Public,” Ecology and Society 5 (2001): 19.
4Lydia Olander, Stephen Polasky, James Kagan, Robert Johnston, Lisa Wainger, David Saah, Lynn Maguire, James Boyd, and David Yoskowitz. “So You Want Your Research to be Relevant? Building the Bridge Between Ecosystem Services Research and Practice,” Ecosystem Services, 26 (2017): 170-182
5 Ringold, P. L., J. Boyd, D. Landers, and M. Weber. “What Data Should We Collect? A Framework for Identifying Indicators of Ecosystem Contributions to Human Well-Being,” Frontiers in Ecology and the Environment 11 (2013): 98–105.
6James Boyd and Alan Krupnick. “Using Ecological Production Theory to Define and Select Environmental Commodities for Nonmarket Valuation,” Agricultural and Resource Economics Review 42, no. 1 (2013): 218-249.